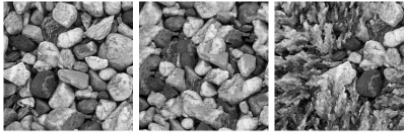
IGWSTQA: Synthesized Texture Quality Assessment via Multi-scale Spatial and Statistical Texture Attributes of Image and Gradient Magnitude Coefficients Magnitudes
In this work we propose a training-free reduced-reference (RR) objective quality assessment method that quantifies the perceived quality of synthesized textures. The proposed reduced-reference synthesized texture quality assessment metric is based on measuring the spatial and statistical attributes of the texture image using both image- and gradient-based wavelet coefficients at multiple scales.
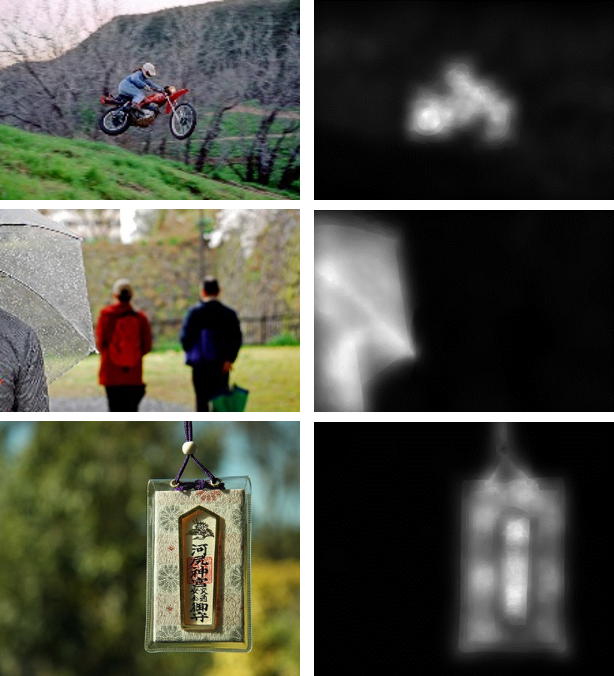
HiFST: Spatially-Varying Blur Detection Based on Multiscale Fused and Sorted Transform Coefficients of Gradient Magnitudes
In this work we have addressed the challenging problem
of blur detection from a single image without having
any information about the blur type or the camera settings.
We proposed an effective blur detection method based on a
high-frequency multiscale fusion and sort transform, which
makes use of high-frequency DCT coefficients of the gradient
magnitudes from multiple resolutions. Our algorithm
achieves state-of-the-art results on blurred images with different
blur types and blur levels.
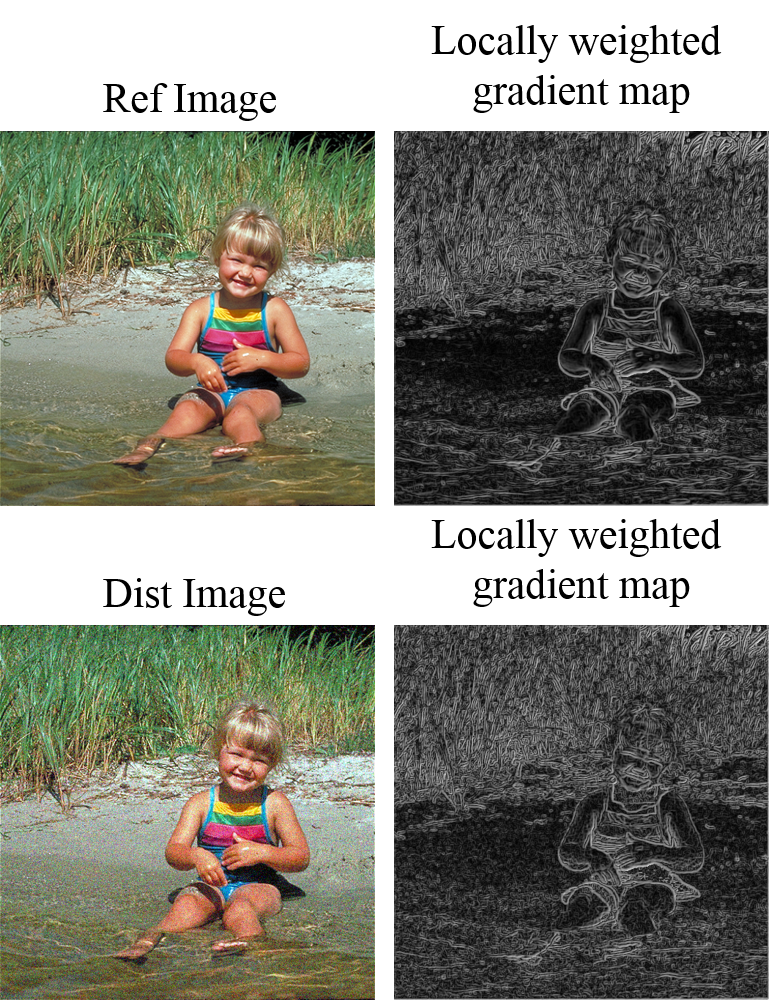
REDLOG: Reduced-Reference Quality Assessment Based on the Entropy of DWT Coefficients of Locally Weighted Gradient Magnitudes
This work presents a training-free low-cost RRIQA method that requires a very small number of RR features (6 RR features).
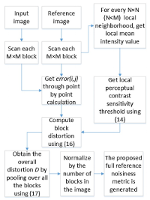
NR_PWN
This work proposes a perceptual based No-reference perceptually weighted noise (NR_PWN) metric by integrating perceptually weighted local noise into a probability summation model. Unlike existing objective metrics, the proposed no-reference metric is able to predict the relative amount of noise perceived in images with different content. Results are reported on both the LIVE and TID2008 databases. The proposed no-reference metric achieves consistently a good performance across noise types and across databases as compared to many of the best very recent no-reference quality metrics.
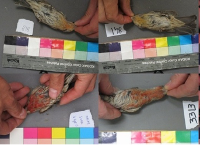
ABCOT
Avian plumage coloration is known to be responsible for many important functions like species identification, camouflage, understanding evolutionary relationships and impact of environmental conditions on habitat and breeding. Bird plumage color quantification refers to the approach of characterizing the plumage coloration observed in individual birds using colorimetric values such as the hue, saturation, brightness,patch size and is an important trait for ecologists. Current color quantification approaches require the user to manually draw a closed contour that encloses the plumage color patch to be analyzed, using an image editing software. Such manual scoring approaches are extremely time consuming and prone to problems like low repeatability and inter observer errors. There is therefore a need for an automated system for plumage color extraction and quantification. The Automated Bird ColOration QuanTification software developed at the IVU lab is a completely automated system that extracts and quantifies plumage coloration in digital images. It has a simple GUI interface that provides the user with different options for storing results and batch processing of images. The various output options include the option to save coloration values in an excel sheet and the option to save an output image that shows a closed contour enclosing the extracted plumage patch in the original image.
ACT
Coloration Quantification has been widely used to study the function and evolution of color signals in animals, birds, reptiles and insects. Current color quantification approaches require the user to manually draw a closed contour that encloses the color patch to be analyzed, using an image editing software. Such manual scoring approaches are extremely time consuming and prone to problems like low repeatability and inter observer errors. There is therefore a need for an automated system for color extraction and quantification. The Automated Coloration quantification software developed at the IVU lab is a completely automated system that extracts and quantifies animal, bird, reptile and insect coloration in digital images. It has a simple GUI interface that provides the user with different options for storing results and batch processing of images. The various output options include the option to save coloration values in an excel sheet and the option to save an output image that shows a closed contour enclosing the extracted color patch in the original image.
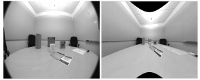
FisheyeCDC Toolbox
Fisheye cameras are special cameras that have a much larger field of view compared to conventional cameras. The large field of view comes at a price of non-linear distortions introduced near the boundaries of the images captured by such cameras. Despite this drawback, they are being used increasingly in many applications of computer vision, robotics, reconnaissance, astrophotography, surveillance and automotive applications. The images captured from such cameras can be corrected for their distortion if the cameras are calibrated and the distortion function is determined. Calibration also allows fisheye cameras to be used in tasks involving metric scene measurement, metric scene reconstruction and other simultaneous localization and mapping (SLAM) algorithms. The FisheyeCDC toolbox incorporates a collection of some of the most widely used techniques for calibration of fisheye cameras under one package with an easy to use interface. This enables an inexperienced user to calibrate his/her own camera without the need for a theoretical understanding about computer vision and camera calibration.
CPDB
This work presents a perceptual-based no-reference objective image sharpness metric (CPBD metric) based on the cumulative probability of blur detection (CPBD). The proposed CPBD metric outperforms existing metrics for Gaussian-blurred and JPEG2000-compressed images.
JNBM
This work presents a perceptual-based no-reference objective image sharpness/blurriness metric by integrating the concept of Just Noticeable Blur (JNB) into a probability summation model. Unlike existing objective no-reference image sharpness/blurriness metrics, the proposed metric is able to predict the relative amount of blurriness in images with different content as well in images with same content. This work received the Best Paper Award by the IEEE Signal Processing Society